ออฟไลน์ด้วยแอป Player FM !
The Promise of Language Models for Search: Generative Information Retrieval
Manage episode 361628091 series 3446693
In this episode of Neural Search Talks, Andrew Yates (Assistant Prof at the University of Amsterdam) Sergi Castella (Analyst at Zeta Alpha), and Gabriel Bénédict (PhD student at the University of Amsterdam) discuss the prospect of using GPT-like models as a replacement for conventional search engines. Generative Information Retrieval (Gen IR) SIGIR Workshop
- Workshop organized by Gabriel Bénédict, Ruqing Zhang, and Donald Metzler https://coda.io/@sigir/gen-ir
- Resources on Gen IR: https://github.com/gabriben/awesome-generative-information-retrieval
References
- Rethinking Search: https://arxiv.org/abs/2105.02274
- Survey on Augmented Language Models: https://arxiv.org/abs/2302.07842
- Differentiable Search Index: https://arxiv.org/abs/2202.06991
- Recommender Systems with Generative Retrieval: https://shashankrajput.github.io/Generative.pdf
Timestamps: 00:00 Introduction, ChatGPT Plugins 02:01 ChatGPT plugins, LangChain 04:37 What is even Information Retrieval? 06:14 Index-centric vs. model-centric Retrieval 12:22 Generative Information Retrieval (Gen IR) 21:34 Gen IR emerging applications 24:19 How Retrieval Augmented LMs incorporate external knowledge 29:19 What is hallucination? 35:04 Factuality and Faithfulness 41:04 Evaluating generation of Language Models 47:44 Do we even need to "measure" performance? 54:07 How would you evaluate Bing's Sydney? 57:22 Will language models take over commercial search? 1:01:44 NLP academic research in the times of GPT-4 1:06:59 Outro
21 ตอน
Manage episode 361628091 series 3446693
In this episode of Neural Search Talks, Andrew Yates (Assistant Prof at the University of Amsterdam) Sergi Castella (Analyst at Zeta Alpha), and Gabriel Bénédict (PhD student at the University of Amsterdam) discuss the prospect of using GPT-like models as a replacement for conventional search engines. Generative Information Retrieval (Gen IR) SIGIR Workshop
- Workshop organized by Gabriel Bénédict, Ruqing Zhang, and Donald Metzler https://coda.io/@sigir/gen-ir
- Resources on Gen IR: https://github.com/gabriben/awesome-generative-information-retrieval
References
- Rethinking Search: https://arxiv.org/abs/2105.02274
- Survey on Augmented Language Models: https://arxiv.org/abs/2302.07842
- Differentiable Search Index: https://arxiv.org/abs/2202.06991
- Recommender Systems with Generative Retrieval: https://shashankrajput.github.io/Generative.pdf
Timestamps: 00:00 Introduction, ChatGPT Plugins 02:01 ChatGPT plugins, LangChain 04:37 What is even Information Retrieval? 06:14 Index-centric vs. model-centric Retrieval 12:22 Generative Information Retrieval (Gen IR) 21:34 Gen IR emerging applications 24:19 How Retrieval Augmented LMs incorporate external knowledge 29:19 What is hallucination? 35:04 Factuality and Faithfulness 41:04 Evaluating generation of Language Models 47:44 Do we even need to "measure" performance? 54:07 How would you evaluate Bing's Sydney? 57:22 Will language models take over commercial search? 1:01:44 NLP academic research in the times of GPT-4 1:06:59 Outro
21 ตอน
ทุกตอน
×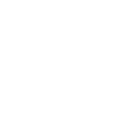
1 The Promise of Language Models for Search: Generative Information Retrieval 1:07:31
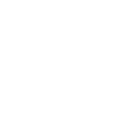
1 Task-aware Retrieval with Instructions 1:11:13
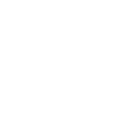
1 Generating Training Data with Large Language Models w/ Special Guest Marzieh Fadaee 1:16:14
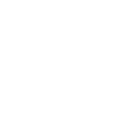
1 Evaluating Extrapolation Performance of Dense Retrieval: How does DR compare to cross encoders when it comes to generalization? 58:30
ขอต้อนรับสู่ Player FM!
Player FM กำลังหาเว็บ